Why Are Migratory Species Harder to Protect Peer Reviewed
Abstruse
Express knowledge of the distribution, abundance, and habitat associations of migratory species hinders effective conservation actions. We employ Neotropical migratory birds as a model group to compare approaches to prioritize land conservation needed to support ≥30% of the global abundances of 117 species. Specifically, we compare scenarios from spatial optimization models to achieve conservation targets by: one) expanse requirements for conserving >30% abundance of each species for each week of the year independently vs. combined; two) including vs. ignoring spatial clustering of species abundance; and three) incorporating vs. avoiding human-dominated landscapes. Solutions integrating data beyond the year require 56% less area than those integrating weekly abundances, with additional reductions when shared-apply landscapes are included. Although incorporating spatial population structure requires more area, geographical representation among priority sites improves substantially. These findings illustrate that globally-sourced citizen science information can elucidate key merchandise-offs among opportunity costs and spatiotemporal representation of conservation efforts.
Introduction
Land-utilize modify is a key threat to the conservation of biodiversity, ecosystemsi, and the services they provide globally2,three, and migratory species are particularly vulnerable to such change given the vast geographic areas they occupy over the almanac bike4,5. Indeed, a recent global assessment indicated that protected areas adequately protect the ranges of but nine% of migratory bird species5. Strategic approaches to identify and conserve habitats disquisitional to the persistence of migratory species are therefore sorely needed.
Unfortunately, substantial gaps in knowledge of the abundance, distribution, and census of most migratory species6 accept hampered strategic planning and led to doubt nigh the optimal resource allotment of conservation effort5,7. Given that populations of many migratory species continue to pass up4,eight, there is an urgent need to place portfolios of lands critical to the persistence of target species, and amenable to direction in support of species conservation without compromising homo well-being.
Multi-species conclusion support tools can facilitate the identification of areas crucial to the conservation of migratory species, simply accept remained intractable due to limits on knowledge and computing power. We capitalized on advances in models of bird species abundance and distribution using crowd-sourced data9,10 and linear programing techniques11 to develop a robust multi-species planning tool to estimate the land area needed to conserve 117 Nearctic-Neotropical migratory songbirds throughout the annual wheel (Supplementary Data 1). Specifically, nosotros combined fine-calibration, predictive models of distribution and abundance estimated weekly throughout the year with spatial optimization techniques12 to place the amount and blazon of country needed to achieve our conservation targets given alternative planning scenarios at hemispheric scales.
Nosotros first estimated the abundance and distribution of 117 migratory bird species weekly, using spatiotemporal exploratory models9,xiii to summate the relative affluence of each species throughout the almanac wheel (Supplementary Movie 1). Incorporating information for each week of the twelvemonth is especially of import for migratory species, as this reflects their movements throughout the annual cycle and allows more precise estimates of their population distributions in space and fourth dimension. We next recorded and compared the geographic area requirements and land cover types selected when optimizing for each week of the year independently and summing the total surface area over all weeks (hereafter, 'weekly'), versus optimizing over the entire year at one time (time to come, 'yearly'). Weekly optimizations for expanse efficiencies were developed to identify species-specific priorities for species at fine plenty scales to capture short-term stopover sites. Our yearly approach optimized efficiency over the full annual cycle of each species, and emphasized temporal consistency in abundance hotspots more likely to reflect breeding and non-breeding regions. Considering all existing conservation plans consider stationary phases of the convenance and non-breeding periods separately14,fifteen, our analysis is the offset instance of spatial optimization scenarios which track populations over their total annual bicycle.
We next created area-optimized solutions designed to conserve lands used by 30% of the global populations of all 117 species in each of 52 weeks by sampling species (a) over their entire range, without accounting for spatial clustering of species abundance, or (b) by sampling within 5 regional population clusters, identified weekly to adjust spatial clustering in species abundance. We wanted to account for the spatial clustering of species abundance because broadly distributed species ofttimes exhibit strong regional-scale variation in abundance across their range. Regional-scale variation in species' abundance may reflect a number of important processes affecting the ecology and conservation of species, from variation in resource availability and land-utilize patterns to population-structure related to move and migratory connectivity. Past accounting for the spatial clustering of species abundance, the prioritization is stratified over multiple regions to ensure adequate protection over the unabridged species range. Because spatial clustering in species abundance—let alone its consequences for movement or connectivity—is poorly understood in most migratory species16, nosotros developed an innovative arroyo to account for structure statistically. Specifically, we used cluster analysis to delineate abundances into 5 spatial clusters of equal abundance and stratified our weekly sampling among clusters to capture the full geographic distribution of each species. Our use of five clusters is an instance that minimized computational endeavour, but which could be extended to optimize by species or goal. Our thirty% target is also arbitrary, but intermediate to the 17% of terrestrial ecosystems targeted by the Convention on Biodiversity17 and 50% targets suggested by comparative analysis18, and can be modified to reflect strategic goals19.
Last, nosotros compared area-based conservation plans designed to represent different perspectives about the potential contribution of homo-modified lands to the conservation of migratory birds. Our 'intact habitat' approach emphasized the protection of relatively intact habitat as indicated by a low human footprint alphabetizetwenty (Supplementary Fig. i), whereas our 'shared-use' approach permitted the inclusion of landscapes converted to more intensive use by humans21,22. Our scenarios, termed intact habitat and permissive of shared-use, are analogous, but more than full general, than state sparing and land sharing scenarios. Exploring such constraints represents a critical pace in conservation planning, given that man cultural history, values, and well-existence can all affect conservation success and represent critical inputs into structured decisions about the almost efficacious deportment23,24,25.
Results
Weekly vs. yearly approaches
The state area required to accomplish yearly conservation targets was 56% less on average than when area targets were summed across weeks (range = 49 to 65%; Table 1). Yearly solutions required relatively less land area than weekly approaches in shared-apply scenarios (62% less) than in intact habitat scenarios (49% less, Table 1, Figs. 1, 2). Surface area reductions nether yearly planning generally resulted from cases such every bit the inclusion of sites used by more 1 species across two or more weeks of the annual cycle. A probable explanation for this difference is that the yearly arroyo will select sites that are used for longer periods of the almanac cycle, and that in that location may exist greater overlap of those areas between species than occurs in the short-term stopover sites included in the weekly approach, hence the larger area needed under the latter.
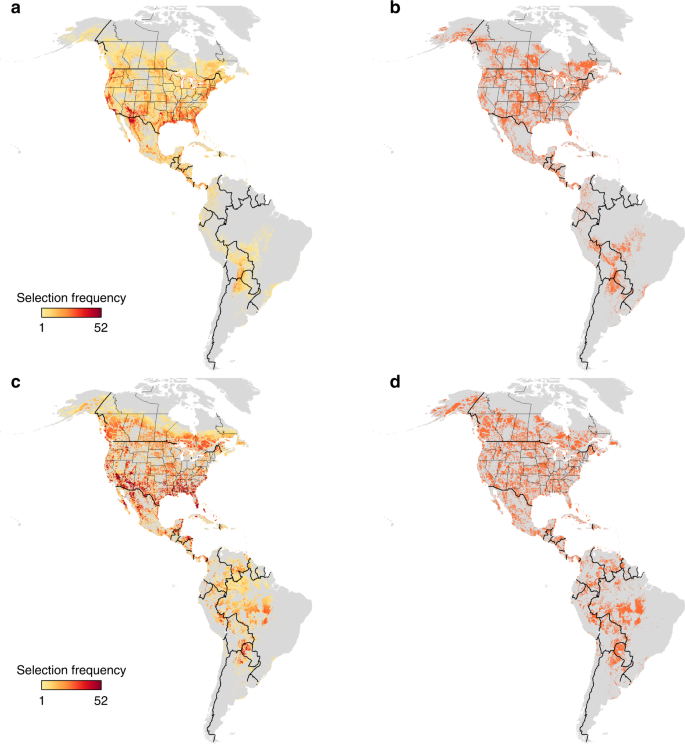
Unmarried population comparison results. Areas prioritized for weekly and yearly planning under a shared-use approach allowing for the inclusion of human being dominated landscapes vs. an intact habitat arroyo that excludes areas of high human footprint are shown. The prioritization is based on a target of 30% of global populations of 117 species of Neotropical migratory birds when each species range is considered as a single population. a = shared-use, weekly, b = shared-use, yearly, c = intact habitat, weekly, d = intact habitat, yearly. Panels a and c testify how frequently (1, light yellow to 52, dark red) areas were selected beyond the weekly solutions. Panels b and d show whether an expanse was selected in a solution ( = red). Supplementary Fig. 2 presents a more detailed version of this figure focusing on northern South America
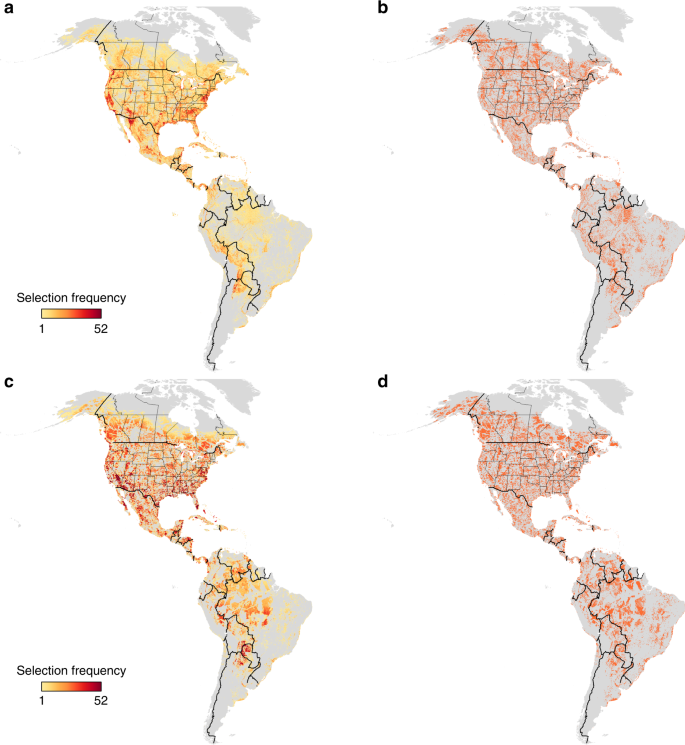
Spatial clustering comparing results. Spatial clustering of species abundance comparison of areas prioritized for weekly and yearly planning under a shared-use approach allowing for the inclusion of human dominated landscapes vs. an intact habitat approach that excludes areas of high human footprint. The prioritization is based on a target of 30% of global populations of 117 species of Neotropical migratory birds when each species range is considered with spatial clustering of species affluence (5 regional clusters). a = shared-utilize, weekly, b = shared-apply, yearly, c = intact habitat, weekly, d = intact habitat, yearly. Panels a and c evidence how often (1, light yellow to 52, dark crimson) areas were selected beyond the weekly solutions. Panels b and d bear witness whether an area was selected in a solution ( = blood-red). Supplementary Fig. 3 presents a more detailed version of this figure focusing on northern South America
Unmarried populations vs. spatial clustering
As expected, the surface area required to reach our conservation targets increased when we accommodated spatial clustering of species abundance, although relatively less so nether a intact habitat (thirteen% increase) compared to a shared-use (26% increase) scenario (Table 1, Figs. 1, two). This reduction occurred in part because the homogenous cost construction used in our intact habitat scenario was less influential on site selection than was the heterogenous cost structure used in the shared-use scenario. Although we currently lack empirical data with which these spatial clusters can be validated, our predictions tin can exist tested direct as tracking and genetic mapping techniques improve to allow comparisons of observed and predicted migration routes. That beingness noted, our electric current method provides a useful approach to ensure geographic representation of spatial clustering of species affluence of a wide suite of species using publicly-available citizen science data in spatial planning tools.
Many conservation interventions, including land protection, are constrained by limits on financial or human resources and the opportunity costs of evolution. Our results show that sampling populations beyond the species range each week required almost twice the amount of land compared to yearly plans based on the relative abundance of species. Our work thus demonstrates the daunting trouble of conserving sufficient land area such that migratory species' dynamic populations are protected throughout the year5,vii,10.
Intact habitat vs. shared-utilize
Another central result of our work is that incorporating conservation objectives in man-dominated habitats may dramatically meliorate the efficiency of conservation area designs for migrants if their demographic performance is similar in 'working' and 'intact' landscapes. Under the yearly scenario we found that shared-use approaches required 26 and 18% less land area, respectively, than intact habitat approaches including or ignoring spatial clustering of species abundance (Table 1). We likewise constitute that intact habitat approaches selected different geographic areas and ecosystems than shared-utilise approaches. Most notably, intact habitat approaches selected larger areas of needle-leaved woods in boreal and mountainous zones of western Canada, and more than wide-leaved evergreen wood in the eastern Andes and western Amazon basin (Figs. 1, two; Tabular array 2). Our findings thus add to a growing body of literature indicating the need to broaden the lens through which we view conservation to both accommodate human livelihoods and conserve species23,24,25.
Overarching considerations
Our findings suggest a need to re-evaluate conservation planning processes that are based on less precise methods. For instance, government and non-governmental organizations allocate up to $1 billion annually to bird conservation based on aspatial targets and proficient elicitation, with most actions directed to breeding habitatfourteen,15. Our results suggest an alternative approach with a potential to meet conservation targets at lower land direction cost and more than compatible with homo-dominated lands that can serve the dual purpose of supporting migratory species and human livelihoods. Overall, our results as well illustrate potential trade-offs that conservation practitioners because optimized portfolios must consider as additional targets and constraints are identified and incorporated in higher-level management models25. Even without consensus among conservation practitioners on focal scenarios, a considerable area of land was selected in at least six of the viii scenarios explored here, illustrating that many priority areas come across a wide range of perspectives encapsulated in our scenarios (126, 000 km^2, Fig. iii). This indicates that even where approaches differ, conservation practitioners tin can use tools like the i described hither to build consensus in hemispheric conservation efforts.
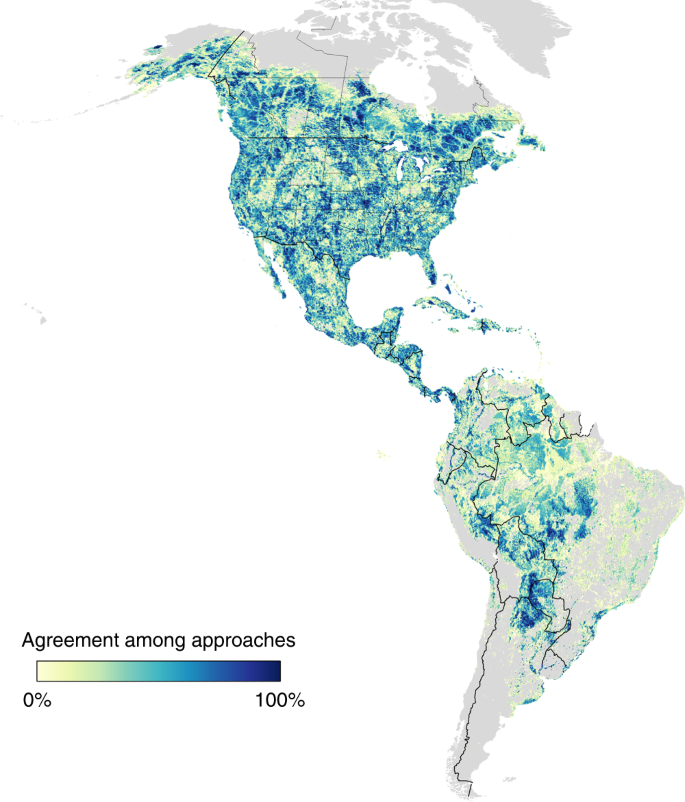
Range of agreement between the eight scenarios investigated. Darker blue indicates that most or all scenarios selected specific areas across the Western Hemisphere, and lighter yellow indicates areas of high scenario specificity. Scenario types considered: (i) summing scenarios for each species in each week of the year vs. optimizing over all weeks and species in a yearly approach, (2) including vs. ignoring spatial clustering of species abundance, and (iii) incorporating vs. avoiding man-dominated landscapes in solutions
Several boosted caveats arise from our results, particularly with respect to the shared-use and intact habitat scenarios. Implementing conservation action in working landscapes may be more than challenging than in areas with less homo activeness if the opportunity costs of management are higher. For instance, even if identified as a high-priority site for conservation in our shared-use scenarios, land already converted to man employ may be more vulnerable to degradation in the futurity than more intact areas26. Such habitat degradation, especially if combined with other anthropogenic stressors that may directly or indirectly reduce survival or performance of wildlife27, could make it difficult to reach population goals for species fifty-fifty if surface area needs are lower compared to less developed landscapes. In practice, both approaches are likely to be used given that target species will differ in their reliance on more or less developed habitat types28. Our prioritization scenarios provide planners with guidance on the approximate locations and requirements for land needed to see our stated targets under a range of scenarios. With such portfolios in hand, planners can then more readily assess the price-effectiveness of alternating approaches to land management and socio-economical policies nigh favorable to conservation and homo well-being23,24,25.
Additional species or landscape characteristics might also be considered in prioritization, depending on the mandate of conservation agencies. For example, the threat status of species has been incorporated into spatial planning recommendations29,30. Our analysis included 19 cherry or yellow-listed species past Partners-in-Flying (Supplementary Data i). In add-on, the specific life-history characteristics and habitat requirements of species, where available, can be important considerations. The 117 species in our analysis varied in spatiotemporal patterns of migration, habitat employ, abundance, and population abundance. Although we did not address how variation in life history amid species might influence results, it is clear that as loftier-resolution distribution models become bachelor for a larger range of migratory species, such as waterfowl, shorebirds, and marine, forest or grassland specialists, planners will have more opportunities to filter species or emphasize particular guilds to represent particular ecosystems or habitats. Different representation targets are besides possible. Nosotros emphasize that the thirty% target used here is illustrative only. In some cases, higher targets may be needed to avert range contraction or the local extinction of sub-populations, to conserve ecological part such as seed dispersal or pest control31, or to maintain the evolutionary potential of locally-adapted populations32,33. Nonetheless, our 30% target returned solutions in all cases which vastly exceed the areal extent of existing conservation plans in support of Neotropical migrant birds.
Discussion
Ongoing declines in the affluence and distribution of many migratory species amid astringent constraints on financial and human resource34 point to an urgent need for expanse-based plans that achieve conservation targets while minimizing the opportunity costs of land conservation and impacts on human livelihood23,24,25,35. Our solutions minimized the total land surface area prioritized for conservation to provide an area-efficient portfolio of lands for farther consideration by conservation planners. Four primal lessons can be derived from our results. First, scenarios based on the distributions of abundance of all 117 species that were integrated over the entire year required less country expanse to meet conservation targets than scenarios based on optimizations that used the weekly distributions of those species throughout the year. This suggests difficult trade-offs betwixt finer-scale spatiotemporal representation of habitats throughout the year, versus solutions that achieve the best compromise on population targets across the entire yr. The latter appears more area-efficient, but may miss primal stopover points forth migratory routes. 2nd, accounting for spatial clustering of species abundance through stratified sampling across the entire distribution of species increased the total land surface area required to reach conservation targets. Despite requiring more land surface area, ensuring geographic representation may be necessary to the long-term persistence of species, particularly in widely-distributed species with population genetic structure potentially reflecting accommodation to local conditions32,33. Third, surface area-based plans that accommodated human being activity (shared-use) were generally more efficient than intact habitat approaches that avoided areas with a high human footprint. Yet, because migrants vary spatially and temporally in their tolerance of human-impacted landscapes36, achieving conservation goals volition likely crave a portfolio of sites located in both intact and disturbed landscapes. Fourth, although our planning scenarios focused on Neotropical migratory birds, our approach could be hands adapted and replicated in other migratory species and systems with sufficient data. In the example of birds, citizen scientific discipline data and advanced prioritization tools allowed united states to reveal marked efficiencies in area-based plans spanning the full annual bike and multiple jurisdictions to conserve 117 individual species simultaneously.
Methods
Species pick
We used the eBird denizen-science database (Sullivan et al., 2014) for this analysis. A total of 224 species were available and we identified a subset of these for assay using the following process. We first examined annual eBird distribution maps for all species to identify Neotropical migratory species (due north = 181 species), divers as those with convenance ranges in North America and not-breeding ranges that extend due south of the Tropic of Cancer37. We and so selected terrestrial passerines from this initial group (n = 117 species, run into Supporting Information Data ane). These 117 species roughshod into two wide groups based on their breeding and stationary not-breeding ranges: (1) species where individuals breed in North America due north of the Usa-Mexico edge and migrate southward of the Tropic of Cancer during the non-convenance flow (n = 101 species, Supplementary Data one), and (2) species with both migratory and resident populations or subspecies, for which individuals from migratory populations north of the US-Mexico border movement south of the Tropic of Cancer during the non-breeding period (n = 16 species). Both migratory and resident populations were included in our analysis.
Approaches to conservation prioritization
Nosotros created 8 planning scenarios using weekly Stem models for each of 117 focal species and incorporating different assumptions most temporal calibration and cost metrics employed in prioritization. First, we created area-optimized solutions to conserve 30% of the global populations of all species past optimizing during each week of the year separately (i.due east., conserving xxx% of populations in each week and summing these private solutions across the year) versus over the entire year (i.e., conserving 30% of total populations calculated in one case throughout the year, run into below and Supplementary Fig. iv for details). We next created area-optimized solutions to conserve xxx% of the global populations of all species in each calendar week by sampling each species a) over their entire range, without bookkeeping for spatial clustering of species affluence, or b) as 5 regional population clusters identified weekly to accommodate spatial clustering of species abundance and migratory connectivity. Third, we compared surface area-based conservation plans designed to represent unlike perspectives about the potential contribution of human-modified landscapes to the conservation of migratory birds, while including either the unrestricted cost metric (each planning unit having the same cost of ane) or the homo footprint cost metric (the planning unit cost equals the 2009, one km resolution human footprint metric by Venter et al.20 to place areas more and less subject to human use), to create a total of viii scenarios (Supplementary Fig. 4). We used the prioritzr38 R package for the analysis, which interfaces with the Gurobi39 optimization software.
Spatial prioritization arroyo
Here we use the concept of systematic conservation planning40, to inform choices about areas to protect, in order to optimize outcomes for biodiversity while minimizing societal costs41. To achieve the goal to optimize the trade-off between conservation benefit and socioeconomic cost, i.e., to get the most benefit for limited conservation funds, we strive to minimize an objective function over a prepare of decision variables, subject to a series of constraints. Integer linear programming (ILP) is the subset of optimization algorithms used hither to solve reserve pattern issues. The full general form of an ILP trouble tin can be expressed in matrix notation equally:
$${\mathrm{Minimize}}\,cx\,{\mathrm{subject}}\,{\mathrm{to}}\,Ax \ge b$$
(1)
where x is a vector of decision variables (in our case, whether to prioritize an individual planning unit), c and b are vectors of known coefficients, and A is the constraint matrix. In the minimum gear up cover problem, c is a vector of costs for each planning unit, b a vector of targets for each conservation feature, the relational operator would be ≥for all features, and A is the representation matrix with Aij =rij, the representation level of feature i in planning unit j. Nosotros set an objective to find the solution that fulfills all the targets and constraints for the smallest area, which we use every bit our measure of costeleven. This objective is like to that used in Marxan, the virtually widely used spatial conservation planning tool42, but has been shown to lead to more efficient solutions11.
Spatiotemporal exploratory models
We used spatiotemporal exploratory models (Stem)9,13,43 to generate estimates of relative abundance for each species. STEM is a type of species distribution model created as an ensemble of local regression models generated from a spatiotemporal cake subsampling design. Repeatedly subsampling and partition the study extent into grids of spatiotemporal blocks, and then fitting independent regression models (base of operations models) in each cake produces an ensemble of partially overlapping local models. Estimates at a given location and date are fabricated past averaging across all the local models that contain the location and appointment. Combining estimates across the ensemble controls for inter-model variability44 and adapts to non-stationary predictor–response relationships13.
The ensemble of spatiotemporal blocks was designed every bit a Monte Carlo sample of 100 randomly located spatiotemporal partitions of the study extent. This results in a uniformly distributed set of spatiotemporal blocks, and up to 100 local models covering each location in the study extent. To account for spatial variation in the density of the bird observation data45, smaller spatiotemporal blocks (10° × 10° × 30 continuous days) were used n of 12° breadth and larger blocks (20° × 20° × 30 continuous days) were used in the southern portion of the written report extent.
The bird observation data used to implement Stalk came from the eBird citizen-science database46. The data included species counts from complete checklists collected nether the 'traveling', 'stationary', and 'areal' protocols from Jan 1, 2004 to December 31, 2016 within the spatial extent divisional past 180° to thirty° West Longitude (likewise every bit Alaska between 150° E and 180° E). This resulted in a dataset consisting of 14 meg checklists collected at 1.vii 1000000 unique locations, of which 10% were withheld for model validation.
Within each spatiotemporal block, species' occupancy and abundance were both assumed to be stationary. If there were at to the lowest degree l checklists with at least 10 detections of the given species inside the spatiotemporal block, we fit a ii-pace additional regression tree model designed to deal with zero-aggrandizementnine to predict the observed counts (affluence). If the minimum sample size requirements were not met for the spatiotemporal cake, that spatiotemporal block was removed from the ensemble. The additional regression trees for both steps of the zero-inflation model were fit with the gbm package47 with bag fraction = 0.lxxx, learning charge per unit or shrinkage = 0.05, and ntrees = 1000. The tree.depth parameter was set to 5 for the occurrence model and x for the abundance model, giving both models the ability to adapt to nonlinear and interacting predictor effects. We relied on the variance-reducing properties from averaging across the Stem ensemble to control for overfitting.
Three general classes of predictors were included in both boosted regression tree models: (i) spatial predictors to account for spatial (and spatiotemporal) patterns; (ii) temporal predictors to account for temporal variation at various scales; and (three) predictors that draw the observation/detection process, which business relationship for variation in detection rates, a nuisance when making inference about species occupancy and abundance.
Spatial data was captured using top48 and NASA MODIS land49 and water comprehend data. All xix cover classes in the MODIS data were summarized within two.eight × two.8 km (784 ha) pixels centered at each eBird location. In each neighborhood, we computed the proportion of each class in the neighborhood (PLAND). To describe the spatial configuration of each class within each neighborhood we computed three statistics using FRAGSTATSfifty and SDMTools51: LPI an index of the largest contiguous patch, PD an index of the patch density, and ED an index of the edge density. Summarizing the land-encompass information at the 2.eight × 2.8 km resolution reduced the impact of erroneous comprehend classifications, and reduced the impact of inaccurate eBird checklist locations. The time of day was used to model variation in availability for detection; e.g., diurnal variation in behavior, such as participation in the 'dawn chorus'52. Day of the year (1-366) was used to capture twenty-four hours-to-solar day changes in occupancy, and year was included to account for year-to-yr differences. Finally, to account for variation in detection rates variables for the number of hours spent searching for species, the length of the transect traveled during the search, and the number of people in the search party were included in each base model.
Relative affluence was estimated as the expected count of birds of a given species on a standardized, hypothetical search conducted by a typical eBird participant starting from the middle of the pixel from vii:00 to 8:00 a.m. while traveling 1 km. Estimates of relative abundance were rendered at weekly temporal resolution and 8.4 × eight.4 km spatial resolution and computed equally the production of the estimated occupancy and, the estimated abundance conditional on occupancy, from the two steps of the boosted regression tree model.
Finally, to ensure good model performance, we required that the ensemble boilerplate computed for each relative abundance estimate have a sample size of at to the lowest degree 50 local models. In preliminary investigations based on practiced review, we found that at least 50 local models were needed to control variation and limit extrapolation.
Weekly and yearly approaches
The two approaches for full annual wheel conservation prioritization used here, were (i) weekly, where one spatial prioritization problem was solved per week and the 52 results where summed together to create a terminal solution where each cell was selected betwixt 0 (i.e., never) to 52 times (i.due east., selected in each solution); (ii) yearly, where the features from each calendar week were included in ane spatial prioritization trouble across all 52 weeks of the year. The solution of this scenario resulted in a cell either being selected or not. For the yearly approach, each week of the yr is its own feature in the optimization. This means, that for a weekly scenario we would accept 117 features (ane for each species) and in the yearly 117*52 features (one for each species in each week of the twelvemonth). To illustrate how the 2 approaches, differ, nosotros have created a theoretical example for ane species and 4 weeks (Supplementary Fig. 5). The example represents a very small report area (forty cells in total vs. four.three million in our bodily assay) and the species has a full of 100 individuals in each week. The target for the species is to protect 30% (i.e., 30 individuals) of the population in each calendar week. The example shows that in full general the weekly approach captures areas important for each calendar week of the year, independent of other weeks of the year. The 'yearly' approach on the other hand optimizes across the year, thereby minimizing expanse requirements, merely as a trade-off it does not necessarily capture the almost important areas in each week of the year.
Sampling for spatial clustering of species abundance
Many of the species used here are represented by multiple sub-species or populations known or suspected to follow unlike migratory pathways and utilise different breeding or wintering habitats5,19,53. Withal, in the absence of detailed noesis on migration pathways for the vast bulk of species, we adult a system of stratified sampling to account for the weekly distribution and spatial structure of each of 117 focal species to ensure representation across their range throughout the almanac cycle. To practise so, we first conducted cluster analyses of predicted weekly distribution maps for all 117 species to identify 5 clusters of equal abundance that encompassed the entire species range to ensure representation across it. We sampled populations as 5 clusters because population structure data was missing for most species, to facilitate a data driven spatial delineation of population centers, and for computational efficiency. Nonetheless, we note that the advisable number of clusters among species may vary past species phylogeography or abundance should sufficient information become bachelor. Our clustering approach re-draws the clusters in each week of the yr, so does not consider whether spatial structure is maintained across weeks—information technology merely imposes a plausible structure inside each week. Our cluster analysis was based on a contrast matrix of geographic locations and abundances (which were weighted by 1/3 to primarily focus on geographic effects and not bias cluster delineation toward spatially separated abundance clusters), and used the CLARA algorithm, which is an extension of the grand-medoids technique for large datasets54. For an instance see Supplementary Fig. 6.
Land use constraints
We used two metrics to constrain our systematic conservation prioritization. First, we used a constant, area-based cost metric for shared-use scenarios, whereby each planning unit of measurement was assigned a cost value of one. In these scenarios, the optimization was driven solely by the species abundance predictions. 2nd, we used homo footprint (2009; one km resolution)20 to identify areas more and less subject area to human use, access or evolution pressures; specifically, we calculated the mean human footprint value for each eight.four × eight.4 km pixel in our study area and used it as the 'cost' of each pixel during prioritization. Nosotros used the man footprint metric as the cost metric in intact habitat scenarios. The human footprint layer represents a blended including the following human pressures: (1) the extent of congenital environments; (2) ingather state; (3) pasture state; (4) human being population density; (v) night-fourth dimension lights; (six) railways; (7) roads; and (8) navigable waterways20. The human footprint values range from 0 to 50, with 0 representing no human pressures and 50 representing the highest human pressure possible. We used the human footprint metric as a continuous constraint in the prioritization approach.
State embrace representation
After the prioritization analyses, we summarized the major state embrace types for each scenario that we generated. We used the 2015 data set up of the global land cover map55 at a 300 m resolution and clipped the original data to the study area. For each scenario, nosotros used the geospatial information abstraction library56 to warp the selected cells from the prioritization onto the raster grid of the state cover dataset. At that place were 37 land cover classes identified beyond scenarios and the frequency and area amount of each was summarized for all scenarios. As a final step nosotros combined similar country cover classes into broader classes (Supplementary Table 2) and nosotros used these to examine differences in surface area and land cover types selected under single flavor vs. full annual cycle planning and for intact habitat vs. shared-utilise scenarios (Table ii).
Reporting summary
Further data on experimental design is available in the Nature Research Reporting Summary linked to this article.
Data availability
All data, computer code used in analysis, files generated from the analysis and outputs such as figures and tables take been deposited and are publicly available here: https://osf.io/58hgs/ (https://doi.org/ten.17605/OSF.IO/58HGS).
References
-
Maxwell, S. 50., Fuller, R. A., Brooks, T. M. & Watson, J. East. M. Biodiversity: the ravages of guns, nets and bulldozers. Nature 536, 143–145 (2016).
-
Bauer, S. & Hoye, B. J. Migratory animals couple biodiversity and ecosystem operation worldwide. Science 344, 1242552 (2014).
-
Semmens, D. J., Diffendorfer, J. Eastward., López-Hoffman, 50. & Shapiro, C. D. Bookkeeping for the ecosystem services of migratory species: quantifying migration support and spatial subsidies. Ecol. Econ. 70, 2236–2242 (2011).
-
Wilcove, D. S. & Wikelski, M. Going, going, gone: is animal migration disappearing. PLoS Biol. 6, e188 (2008).
-
Runge, C. A., Martin, T. G., Possingham, H. P., Willis, S. Thousand. & Fuller, R. A. Conserving mobile species. Front. Ecol. Environ. 12, 395–402 (2014).
-
Zuckerberg, B., Fink, D., La Sorte, F. A., Hochachka, W. M. & Kelling, Due south. Novel seasonal land cover associations for eastern Northward American forest birds identified through dynamic species distribution modelling. Divers. Distrib 22, 717–730 (2016).
-
Runge, C. A., Tulloch, A. I. T., Possingham, H. P., Tulloch, Five. J. D. & Fuller, R. A. Incorporating dynamic distributions into spatial prioritization. Divers. Distrib 22, 332–343 (2016).
-
Harris, G., Thirgood, S., Hopcraft, J., Cromsight, J. & Berger, J. Global decline in aggregated migrations of large terrestrial mammals. Endanger. Species Res. 7, 55–76 (2009).
-
Johnston, A. et al. Affluence models improve spatial and temporal prioritization of conservation resources. Ecol. Appl. 25, 1749–1756 (2015).
-
La Sorte, F. A. et al. Global change and the distributional dynamics of migratory bird populations wintering in Cardinal America. Global Change Biol. (2017). https://doi.org/x.1111/gcb.13794
-
Beyer, H. Fifty., Dujardin, Y., Watts, M. E. & Possingham, H. P. Solving conservation planning problems with integer linear programming. Ecol. Modell. 328, 14–22 (2016).
-
Moilanen, A., Wilson, K. A. & Possingham, H. P. Spatial conservation prioritization: quantitative methods and computational tools. Vol. 6 (Oxford Academy Press Oxford, UK, 2009).
-
Fink, D. et al. Spatiotemporal exploratory models for broad-calibration survey data. Ecol. Appl. 20, 2131–2147 (2010).
-
Species At Chance Act. Bill C-5, An act respecting the protection of wild fauna species at risk in Canada. (2002).
-
U.Southward. Congress. Endangered Species Deed of 1973 (xvi U.S.C. 1531–1544, 87 Stat. 884). (1973).
-
Webster, M. Southward., Marra, P. P., Haig, South. Yard., Bensch, Southward. & Holmes, R. T. Links between worlds: unraveling migratory connectivity. Trends Ecol. Evol. 17, 76–83 (2002).
-
MacKinnon, D. et al. Canada and Aichi Biodiversity Target 11: understanding 'other effective expanse-based conservation measures' in the context of the broader target. Biodivers. Conserv. 24, 3559–3581 (2015).
-
Noss, R. F. et al. Bolder thinking for conservation. Conserv. Biol. 26, ane–4 (2012).
-
Wilson, Southward. et al. Prioritize multifariousness or declining species? Trade-offs and synergies in spatial planning for the conservation of migratory birds. Preprint at https://www.biorxiv.org/content/x.1101/429019v1 (2018).
-
Venter, O. et al. Sixteen years of modify in the global terrestrial human footprint and implications for biodiversity conservation. Nat. Commun. vii, 12558 (2016).
-
Phalan, B., Onial, M., Balmford, A. & Light-green, R. E. Reconciling food production and biodiversity conservation: land sharing and state sparing compared. Science 333, 1289–1291 (2011).
-
Kremen, C. & Merenlender, A. Chiliad. Landscapes that piece of work for biodiversity and people. Scientific discipline 362, eaau6020 (2018).
-
Barrett, C. B. & Arcese, P. Are integrated conservation-development projects (ICDPs) sustainable? On the conservation of big mammals in sub-Saharan Africa. World Dev. 23, 1073–1084 (1995).
-
Ban, N. C. et al. A social–ecological approach to conservation planning: embedding social considerations. Front. Ecol. Environ. eleven, 194–202 (2013).
-
Schwartz, M. W. et al. Decision support frameworks and tools for conservation. Conserv. Lett. 11, e12385 (2018).
-
Haddad, N. Yard. et al. Habitat fragmentation and its lasting bear upon on Earth'southward ecosystems. Sci. Adv. 1, (2015).
-
Loss, Due south. R., Will, T. & Marra, P. P. Straight mortality of birds from anthropogenic causes. Ann. Rev. Ecol. Evol. Syst. 46, 99–120 (2015).
-
Jenkins, C. North., Pimm, S. Fifty. & Joppa, L. N. Global patterns of terrestrial vertebrate diversity and conservation. Proc. Natl Acad. Sci. 110, E2602–E2610 (2013).
-
Butchart, Southward. H. M. et al. Shortfalls and solutions for meeting national and global conservation area targets. Conserv. Lett. eight, 329–337 (2015).
-
Venter, O. et al. Targeting global protected area expansion for imperiled biodiversity. PLoS Biol. 12, e1001891 (2014).
-
Kenis, M., Hurley, B. P., Hajek, A. Eastward. & Cock, Chiliad. J. W. Classical biological control of insect pests of trees: facts and figures. Biol. Invasions 19, ane–17 (2017).
-
Bay, R. A. et al. Genomic signals of selection predict climate-driven population declines in a migratory bird. Scientific discipline 359, 83–86 (2018).
-
Arcese, P. & Keller, Fifty. Population Construction. in Ornithology: Foundation, Assay, and Awarding (eds. Morrison, One thousand. 50., Rodewald, A. D., Voelker, G., Colón, M. R. & Prather, J. F.) (JHU Press, Baltimore Maryland, The states 2018).
-
McCarthy, D. P. et al. Financial costs of meeting global biodiversity conservation targets: current spending and unmet needs. Science 338, 946–949 (2012).
-
Bottrill, One thousand. C. et al. Is conservation triage just smart decision making? Trends Ecol. Evol. 23, 649–654 (2008).
-
Faaborg, J. et al. Recent advances in understanding migration systems of New World state birds. Ecol. Monogr. fourscore, 3–48 (2010).
-
Hagan Iii, J. M. & Johnston, D. West. Environmental and conservation of Neotropical migrant landbirds. In Symposium on Environmental and Conservation of Neotropical Migrant Country birds 6-ix Dic 1989 Woods Hole, Mass. (EUA) (Smithsonian Institution Printing, Washington, DC, USA 1991).
-
Hanson, J. et al. prioritizr: Systematic Conservation Prioritization in R, Version 3.0.three. (2017).
-
Gurobi Optimization Inc. Gurobi Optimizer Reference Transmission, Version 7.5.ane. (2017).
-
Margules, C. R. & Pressey, R. L. Systematic conservation planning. Nature 405, 243–253 (2000).
-
McIntosh, E. J., Pressey, R. L., Lloyd, Southward., Smith, R. & Grenyer, R. The bear upon of systematic conservation planning. Ann. Rev. Environ. Res. 42, 102016-060902 (2017).
-
Ball, I. R. R., Possingham, H. P. P. & Watts, M. Due east. E. Marxan and relatives: software for spatial conservation prioritisation. In Spatial conservation prioritisation: quantitative methods and computational tools. (eds. Moilanen, A., Wilson, K. & Possingham, H. P.) 185–195 (Oxford Academy Printing, 2009).
-
Fink, D. et al. Crowdsourcing meets ecology: hemisphere-wide spatiotemporal species distribution models. AI Magazine 35, 19–xxx (2014).
-
Efron, B. Interpretation and accuracy later on model pick. J. Am. Stat. Assoc. 109, 991–1007 (2014).
-
Fink, D., Damoulas, T. & Dave, J. Adaptive Spatio-Temporal Exploratory Models: Hemisphere-wide species distributions from massively crowdsourced eBird information. (AAAI, Bellevue, Washington, USA 2013).
-
Sullivan, B. Fifty. et al. The eBird enterprise: an integrated arroyo to development and application of citizen scientific discipline. Biol. Conserv. 169, 31–40 (2014).
-
Ridgeway, M. gbm: Generalized Boosted Regression Models. R parcel. (2017).
-
Amatulli, G. et al. A suite of global, cantankerous-scale topographic variables for environmental and biodiversity modeling. Sci. Data v, 180040 (2017).
-
Friedl, M. A. et al. MODIS Collection five global country encompass: algorithm refinements and label of new datasets. Remote Sens. Environ. 114, 168–182 (2010).
-
McGarigal, Grand., Cushman, S. A. & Ene, E. FRAGSTATS v4: spatial pattern analysis program for categorical and continuous maps. University of Massachusetts, Amherst, Massachusetts, USA. goo. gl/aAEbMk (2012).
-
VanDerWal, J., Falconi, L., Januchowski, S., Shoo, L. & Storlie, C. SDMTools: Species Distribution Modelling Tools: Tools for processing information associated with species distribution modelling exercises. R package version ane (2014).
-
Diefenbach, D. R., Marshall, Chiliad. R., Mattice, J. A. & Brauning, D. Due west. Incorporating availability for detection in estimates of bird abundance. Auk 124, 96–106 (2007).
-
Martin, T. 1000. et al. Optimal conservation of migratory species. PLoS 1 2, 751 (2007).
-
Kaufman, L. & Rousseeuw, P. J. Sectionalization around medoids (plan pam). Finding groups in data: an introduction to cluster analysis. 344, 68–125 (1990).
-
ESA Climate change Initiative. Global land cover map 300m resolution for 2015. http://maps.elie.ucl.ac.exist/CCI/viewer/download.php. (2017).
-
Warmerdam, F. The geospatial data brainchild library. In Open source approaches in spatial data handling, 87–104 (Springer, Berlin Heidelberg, Deutschland 2008).
Acknowledgements
R.South. is supported by a Liber Ero Fellowship and Surround and Climate Modify Canada (ECCC), A.D.R. by a Garvin endowment, and P.A. past the Natural Sciences and Engineering Research Quango of Canada (NSERC), and J.R.B. by NSERC and ECCC. We too thank the eBird participants for their contributions and eBird team for their support. This work was funded by The Leon Levy Foundation, The Wolf Creek Charitable Foundation, NASA (NNH12ZDA001N-ECOF), Microsoft Azure Research Honour (CRM: 0518680), and the National Science Foundation (ABI sustaining: DBI-1356308; calculating support from CNS-1059284 and CCF-1522054).
Writer data
Affiliations
Contributions
R.S., S.W., A.D.R., J.R.B. and P.A. conceived the study. R.South., D.F. and T.A. nerveless information and conducted analyses. All authors contributed to writing and editing the paper.
Corresponding author
Ideals declarations
Competing interests
The authors declare no competing interests.
Additional information
Journal peer review data: Nature Communications thank you the anonymous reviewers for their contribution to the peer review of this work. Peer reviewer reports are available
Publisher's note: Springer Nature remains neutral with regard to jurisdictional claims in published maps and institutional affiliations.
Supplementary information
Rights and permissions
Open up Access This commodity is licensed nether a Artistic Eatables Attribution iv.0 International License, which permits use, sharing, adaptation, distribution and reproduction in any medium or format, as long as you give appropriate credit to the original writer(south) and the source, provide a link to the Artistic Commons license, and betoken if changes were made. The images or other third party material in this article are included in the commodity's Creative Commons license, unless indicated otherwise in a credit line to the material. If material is not included in the article's Artistic Eatables license and your intended use is not permitted by statutory regulation or exceeds the permitted apply, you will need to obtain permission directly from the copyright holder. To view a copy of this license, visit http://creativecommons.org/licenses/by/four.0/.
Reprints and Permissions
About this article
Cite this commodity
Schuster, R., Wilson, S., Rodewald, A.D. et al. Optimizing the conservation of migratory species over their total annual cycle. Nat Commun ten, 1754 (2019). https://doi.org/x.1038/s41467-019-09723-eight
-
Received:
-
Accepted:
-
Published:
-
DOI : https://doi.org/10.1038/s41467-019-09723-eight
Farther reading
Comments
By submitting a comment you concur to abide by our Terms and Customs Guidelines. If you find something abusive or that does not comply with our terms or guidelines please flag it as inappropriate.
Source: https://www.nature.com/articles/s41467-019-09723-8
0 Response to "Why Are Migratory Species Harder to Protect Peer Reviewed"
ارسال یک نظر